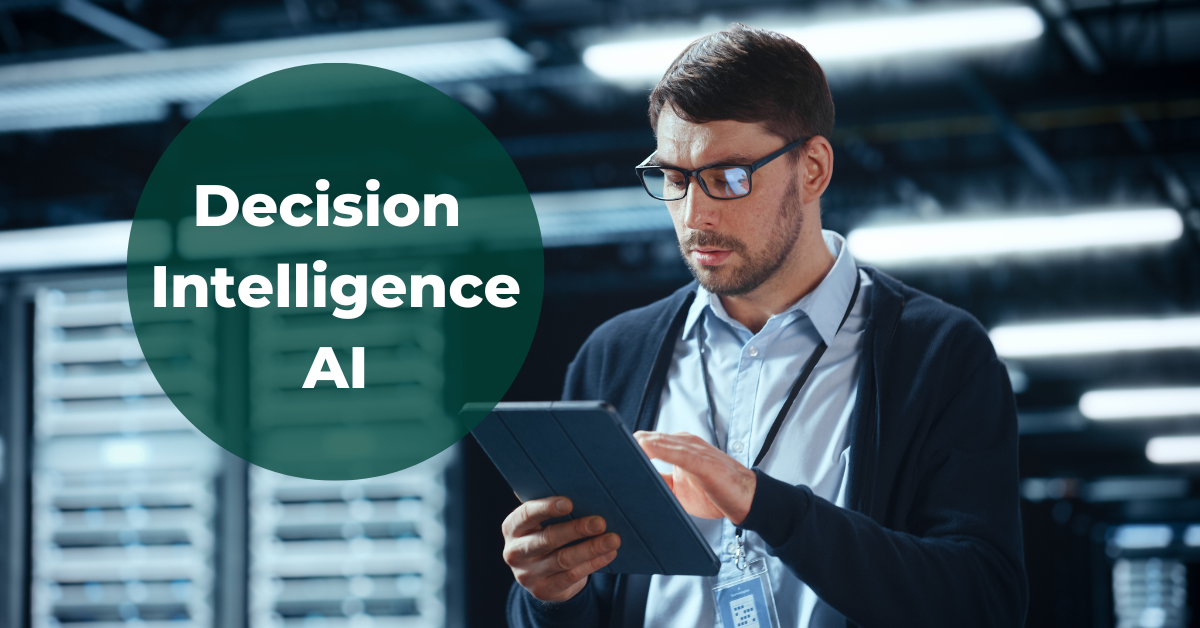
What are the steps included in data integration processes?
The data integration process is a crucial aspect of modern data management, enabling organizations to consolidate and harmonize data from various sources into a unified and coherent format. This process involves several key steps aimed at ensuring the seamless flow of data across different systems and platforms. In this article, we’ll explore the fundamental steps included in the data integration process and how they contribute to the efficient management and utilization of data within an organization.
The data integration process involves several key steps to ensure the successful consolidation, transformation and harmonization of data from diverse sources. Here are the essential steps typically included in a data integration process:
- Requirements Gathering: Understand the organization’s data integration needs, identify data sources, formats, and specific requirements.
- Data Profiling: Analyze source data for quality issues, inconsistencies, and anomalies.
- Data Extraction: Extract data using methods like batch processing or real-time streaming.
- Data Transformation: Standardize and clean extracted data to align with business requirements.
- Data Mapping and Matching: Map data elements from source to target systems, resolving inconsistencies.
- Data Loading: Load transformed data into the target repository or warehouse while maintaining integrity.
- Data Verification and Validation: Validate integrated data for quality, accuracy, and completeness.
- Data Governance and Security: Implement policies and measures to protect data privacy and ensure compliance.
What are the types of data integration
- Batch Data Integration: Involves processing data in large batches at scheduled intervals. Data is extracted from source systems, transformed, and loaded into the target system in predefined batches.
- Real-Time Data Integration: Involves processing data in near real-time or in real-time as it becomes available. Changes to source data are immediately captured, transformed, and propagated to the target system in real-time.
- Cloud Data Integration: Involves integrating data from cloud-based sources, such as SaaS applications, cloud databases, and cloud storage services, with on-premises or other cloud-based systems.
- Enterprise Application Integration (EAI): Involves integrating data and processes across different enterprise applications, such as ERP systems, CRM systems, and legacy systems, to enable seamless communication and data sharing.
- Data Warehousing: Involves integrating data from multiple sources into a centralized data warehouse or data lake, where it can be stored, organized, and analyzed for reporting and analytics purposes.
- Data Virtualization: Involves creating a virtual layer that abstracts and integrates data from multiple sources in real-time, providing users with a unified view of the data without physically moving or storing it.
What are examples of data integration
- Customer Relationship Management (CRM) Integration: Integrating customer data from CRM systems with marketing automation platforms to create targeted marketing campaigns based on customer insights.
- E-commerce Integration: Integrating product data from inventory management systems with e-commerce platforms to ensure accurate product listings, pricing, and inventory availability.
- Healthcare Integration: Integrating patient data from electronic health records (EHR) systems with diagnostic systems and medical devices to provide clinicians with real-time patient information and insights.
What is an example of a data integration strategy?
An example of a data integration strategy is implementing a real-time data integration solution to synchronize customer data across multiple systems in a retail organization. This strategy involves:
- Identifying Data Sources: Identify the various systems and applications that store customer data, such as CRM systems, POS systems, and e-commerce platforms.
- Selecting Integration Tools: Select appropriate integration tools and technologies, such as ETL (Extract, Transform, Load) tools, data integration platforms, and APIs, to facilitate real-time data synchronization.
- Implementing Data Pipelines: Implement data pipelines to capture changes to customer data in real-time, transform the data as needed, and propagate the changes to the target systems.
- Ensuring Data Quality: Implement data quality checks and validation rules to ensure the accuracy, completeness, and consistency of the integrated customer data.
- Monitoring and Maintenance: Monitor the data integration processes in real-time to detect any issues or anomalies, and perform regular maintenance tasks to optimize performance and ensure data integrity.
Data integration is essential for organizations seeking to harness the full potential of their data. By combining data from diverse sources, organizations can achieve a unified view that drives informed decision-making and operational efficiency. Successful data integration not only enhances data quality but also enables seamless access to comprehensive insights, fostering better business outcomes. Effective data integration transforms raw data into valuable insights, empowering organizations to make strategic, data-driven decisions that enhance their competitive edge and operational performance.