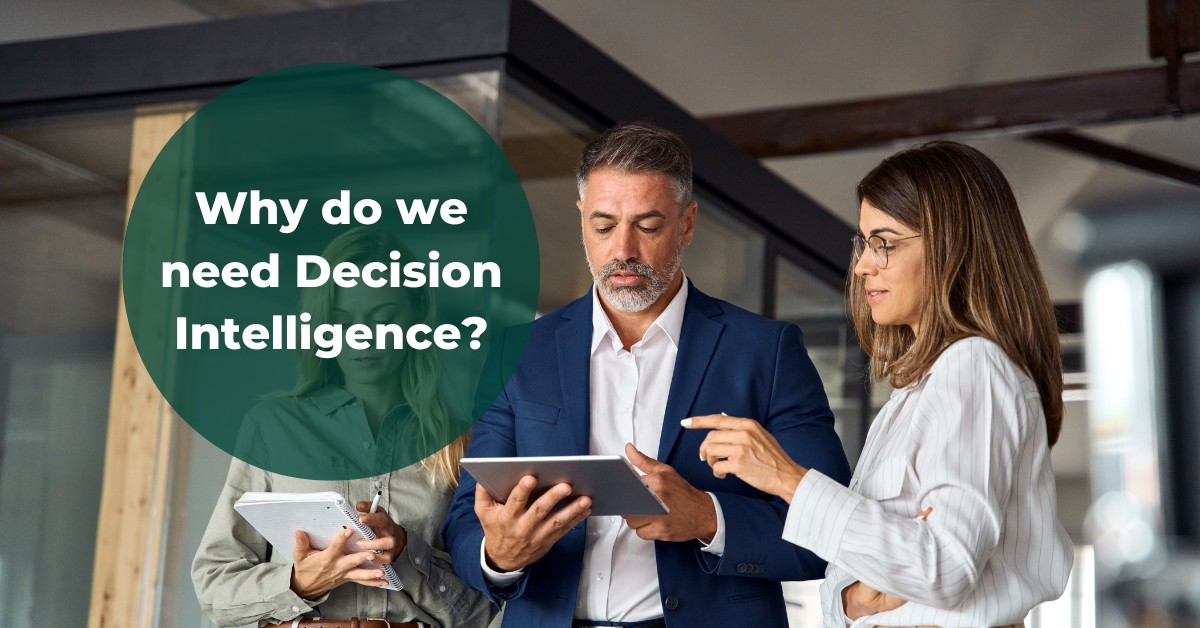
Decision Intelligence in AI
In the intricate realm of Artificial Intelligence (AI), the concept of Decision Intelligence (DI) emerges as a critical component, showcasing the maturation of AI systems beyond mere data processing. Decision intelligence in AI signifies a sophisticated approach aimed at elevating decision-making processes to new heights, blending computational efficiency with human-like discernment.
Advancements in artificial intelligence have profoundly transformed decision-making, enabling the proficient analysis of intricate data and variables in complex scenarios. This capability accelerates and enhances decision-making for companies compared to scenarios where humans tackle challenges unaided. However, it’s crucial to recognize that, despite AI’s prowess in data processing, it lacks the inherent human qualities of wisdom and discernment. Human involvement is therefore essential. The goal of integrating AI into decision-making is not complete automation but augmentation—enabling humans to make faster and improved decisions through efficient data utilization and streamlined processes. This discussion delves into AI’s multifaceted role in decision-making, addressing challenges encountered during integration and presenting real-world examples. By exploring these facets, we aim to provide insights into the transformative potential that AI holds for teams and businesses.
Importance of AI in Decision-Making
Artificial intelligence plays a pivotal role in decision-making across various industries, revolutionizing the way organizations analyze data, derive insights and implement strategies. The importance of AI in decision-making can be explained through several key aspects:
Data Processing and Analysis: AI systems excel at processing vast amounts of data at unprecedented speeds, enabling organizations to make decisions based on comprehensive and real-time information. Machine learning algorithms can identify patterns, trends and correlations within data, providing valuable insights that may not be apparent through traditional analytical methods.
Predictive Analytics: AI facilitates predictive modeling by analyzing historical data and identifying future trends. This capability assists decision-makers in forecasting outcomes, enabling proactive and strategic decision-making. Businesses can use predictive analytics to anticipate market trends, customer behavior and potential risks, allowing them to stay ahead of the competition.
Automation and Efficiency: AI automates routine and repetitive tasks, freeing up human resources to focus on more complex and creative aspects of decision-making. Automation enhances efficiency by reducing the likelihood of human error and streamlining processes, leading to quicker and more accurate decision outcomes.
Personalization and Customer Experience: AI-powered recommendation systems enhance customer experiences by analyzing user preferences and behaviors. This enables businesses to tailor products, services, and marketing strategies to individual customer needs. The ability to personalize interactions contributes to increased customer satisfaction and loyalty.
Risk Management: AI assists in identifying and mitigating risks by analyzing data patterns associated with potential threats or challenges. In finance, for example, AI models can analyze market conditions and detect anomalies, providing early warnings about potential financial risks.
Strategic Planning: AI aids in strategic decision-making by providing data-driven insights that support the development of long-term plans. Businesses can use AI to simulate different scenarios, allowing decision-makers to assess the potential impact of various strategies and make informed choices.
Healthcare Decision Support: In healthcare, AI contributes to clinical decision support systems by analyzing patient data, medical records, and research findings. This assists healthcare professionals in making more accurate diagnoses and treatment plans.
Supply Chain Optimization: AI helps optimize supply chain operations by forecasting demand, managing inventory, and identifying areas for cost savings. This is crucial for organizations seeking to improve overall efficiency and reduce operational costs.
Continuous Learning and Adaptation: AI systems can continuously learn and adapt to changing circumstances. This adaptability is crucial in dynamic environments where decisions must be adjusted in response to evolving conditions.
Challenges in Utilizing AI for Decision-Making
Artificial intelligence brings unprecedented advantages to decision-making processes, yet its integration is not without challenges. Understanding and addressing these challenges is essential for harnessing the full potential of AI in decision-making.
Data Quality and Bias: The quality of AI-driven decisions heavily depends on the accuracy and relevance of the data it processes. Biases present in training data can lead to skewed outcomes, impacting the fairness and objectivity of decision-making processes.
Interpretability and Explainability: AI models, particularly complex ones like deep neural networks, often lack transparency in their decision-making processes. The inability to explain how AI arrives at a specific decision poses challenges, especially in sectors where transparency and accountability are paramount.
Ethical Implications: AI systems may inadvertently perpetuate or amplify existing societal biases. Ensuring that AI decisions align with ethical standards and do not discriminate against certain groups is an ongoing challenge that requires careful scrutiny and regulatory frameworks.
Integration with Human Decision-Makers: Successfully integrating AI into decision-making processes requires collaboration between AI systems and human decision-makers. Resistance to change or a lack of understanding about AI capabilities can hinder the seamless incorporation of AI insights into the decision-making workflow.
Security Concerns: As AI systems become more prevalent, they become potential targets for malicious activities. Safeguarding AI systems from cyber threats and ensuring the integrity of decision-making processes are critical challenges in the implementation of AI.
Limited Generalization: AI models trained on specific datasets may struggle to generalize well to new or unseen scenarios. This limitation can impact the reliability of AI-driven decisions, particularly in dynamic environments where conditions may evolve.
Resource Intensiveness: Training and maintaining sophisticated AI models can be resource-intensive in terms of computing power, time and expertise. Smaller organizations or those with limited resources may face challenges in adopting and sustaining advanced AI solutions for decision-making.
Regulatory Compliance: Adhering to data protection and privacy regulations while utilizing AI in decision-making is a complex challenge. Striking a balance between leveraging the benefits of AI and complying with stringent regulatory requirements requires careful navigation.
Overreliance on AI: Blindly relying on AI without human oversight can lead to complacency and errors. Establishing a balance where AI augments human decision-making without replacing critical human judgment is a challenge that organizations must carefully navigate.
Influence of Artificial Intelligence in Decision Making Process
Artificial intelligence is being employed across diverse sectors to significantly impact decision-making processes through a range of innovative applications. The integration of AI into decision-making can be observed in various facets, each contributing to enhanced efficiency and effectiveness.
Data Analysis and Pattern Recognition:
Data analysis and pattern recognition play a pivotal role in the realm of artificial intelligence decision-making, serving as the bedrock upon which intelligent systems derive meaningful insights from vast and complex datasets. The synergy between data analysis and pattern recognition empowers AI models to discern trends, relationships and anomalies, thereby contributing to more informed and effective decision-making processes across a myriad of industries.
- Comprehensive Understanding through Data Analysis: At the core of AI decision-making lies the ability to process and analyze extensive datasets. Data analysis within the AI framework involves the systematic examination of raw information to uncover trends, correlations and statistical significance. This comprehensive understanding of data forms the basis for informed decision-making.
- Identifying Patterns and Trends: One of the primary strengths of AI lies in its capacity to recognize patterns within data. Machine learning algorithms, a subset of AI, excel at identifying complex relationships and trends that may elude traditional analytical approaches. This ability is crucial for decision-makers seeking to extract valuable insights from large and diverse datasets.
- Extraction of Relevant Features: Through advanced data analysis techniques, AI systems can discern relevant features within datasets. This involves identifying the variables and attributes that contribute most significantly to a particular outcome or trend. This feature extraction is essential for creating models that focus on the most relevant aspects of the data.
- Real-time Data Processing: In dynamic environments, the ability to process data in real-time is paramount. AI systems, equipped with robust data analysis capabilities, can handle streaming data and make decisions on the fly. This real-time processing ensures that decision-makers have access to the most up-to-date information, enhancing the agility of the decision-making process.
- Statistical Inference: Data analysis in AI involves statistical techniques that enable the system to make inferences about a population based on a sample of data. This statistical reasoning enhances the reliability of decisions by providing a quantifiable measure of confidence in the conclusions drawn from the data.
- Anomaly Detection: Through the recognition of patterns, AI systems can identify anomalies or outliers within datasets. Anomaly detection is crucial for flagging unusual patterns that may indicate errors, fraud or other irregularities. This contributes to risk management and ensures the integrity of decision-making processes.
Predictive Analytics:
Predictive analytics, a pivotal component of artificial intelligence in decision-making, revolutionizes the strategic landscape by leveraging advanced algorithms to forecast future trends, outcomes and behaviors. This innovative application of AI not only empowers organizations to make proactive decisions but also plays a fundamental role in optimizing resource allocation and risk mitigation.
- Historical Data Insight: Predictive analytics relies on historical data to discern patterns and correlations. By analyzing past trends and events, AI systems develop a contextual understanding that serves as the foundation for predicting future occurrences.
- Algorithmic Forecasting: AI employs sophisticated machine learning algorithms to identify and extrapolate patterns from historical datasets. These algorithms adapt and evolve, continuously refining their predictive capabilities as they encounter new data, making them adept at handling dynamic scenarios.
- Anticipating Trends and Opportunities: The primary goal of predictive analytics in AI is to anticipate trends and identify opportunities before they manifest. Organizations can harness this foresight to capitalize on emerging market trends, customer preferences and potential opportunities, thereby gaining a competitive edge.
- Proactive Decision-Making: Unlike traditional decision-making, which is often reactive, predictive analytics enables a proactive approach. By foreseeing potential outcomes, organizations can strategize and make decisions well in advance, mitigating risks and capitalizing on favorable conditions.
Automation for Efficiency:
Automation in artificial intelligence is a cornerstone of efficiency in decision-making, streamlining processes, minimizing manual intervention, and accelerating the overall workflow. This symbiotic integration of automation and AI not only enhances efficiency but also reduces errors, allowing decision-makers to focus on strategic aspects and complex problem-solving. Here’s a breakdown of how automation contributes to efficiency in AI-driven decision-making:
- Routine Task Handling: AI excels at automating routine and repetitive tasks that would otherwise consume valuable human resources. Whether it’s data entry, report generation, or basic computations, automation frees up time for decision-makers to concentrate on tasks that require strategic thinking and creativity.
- Data Processing Speed: Automation, coupled with AI, significantly increases the speed at which vast amounts of data can be processed. This rapid data processing capability ensures that decision-makers have access to real-time information, facilitating quicker responses to dynamic situations.
- Algorithmic Decision Execution: Automated decision execution is a hallmark of AI, where algorithms make decisions based on predefined rules and criteria. This not only expedites the decision-making process but also ensures consistency and adherence to specified guidelines.
- Workflow Optimization: Automation optimizes end-to-end workflows by seamlessly integrating AI into existing systems. Decision-making processes benefit from streamlined workflows, reducing bottlenecks and enhancing the overall efficiency of operations.
- Error Reduction: Human error is inherent in manual processes. Automation mitigates this risk by executing tasks with precision and consistency. In decision-making, the reduction of errors is crucial for maintaining the integrity of analyses and ensuring that conclusions are based on accurate information.
- Resource Allocation Optimization: AI-driven automation assists in optimizing resource allocation. Whether it’s allocating budget resources, personnel, or other assets, automated systems can analyze data and make recommendations, ensuring that resources are directed where they are most needed.
Natural Language Processing (NLP):
Natural Language Processing (NLP) is a transformative force within the landscape of artificial intelligence, especially in the domain of decision intelligence. Its impact is most pronounced in the realm of communication, where it facilitates a seamless interaction between humans and AI systems. Unlike conventional decision-making processes limited to structured data, NLP enables AI systems to comprehend and interpret unstructured data sources, such as text and speech. This newfound ability to understand the nuances of human language fosters enhanced human-machine interaction, making communication more natural and intuitive.
Conversational AI, empowered by NLP, emerges as a powerful tool in decision-support scenarios. Users can engage in meaningful dialogues with AI systems, articulating complex queries, receiving detailed responses and refining their requests iteratively. NLP not only interprets the meaning of words but also excels in contextual understanding, ensuring accurate interpretation and response generation based on the surrounding context. A notable application of NLP in decision intelligence is the extraction of insights from textual data. Through techniques like sentiment analysis and entity recognition, NLP allows AI systems to distill meaningful information from text, providing decision-makers with a comprehensive understanding of context and sentiment.
As NLP-driven systems interact with users, they exhibit adaptive learning, continuously improving their language understanding over time. This adaptability enhances the accuracy and relevance of AI-driven decision support, making the integration of NLP a crucial element in shaping the future of decision intelligence within the evolving landscape of artificial intelligence.
Decision Intelligence Algorithm in AI
Decision Intelligence (DI) algorithms in artificial intelligence play a pivotal role in enhancing the decision-making capabilities of systems. These algorithms are designed to process complex data sets, identify patterns and generate insights that can aid in making informed and effective decisions. Some of the algorithms used in AI for decision-making are:
Decision Tree Algorithms: Decision trees are popular for their simplicity and interpretability. They work by recursively splitting the dataset based on feature values, creating a tree structure. Each leaf node represents a decision or outcome. Decision trees are versatile and widely used in decision intelligence for tasks like classification and regression.
Random Forest: Random Forest is an ensemble learning technique that builds multiple decision trees and merges their outputs. This approach enhances accuracy and reduces overfitting. In decision intelligence, Random Forests are employed to handle complex decision-making scenarios by combining the strengths of multiple trees.
Support Vector Machines (SVM): SVM is effective for classification tasks in decision intelligence. It finds the hyperplane that best separates data points into different classes. SVMs are particularly useful when dealing with high-dimensional data, making them applicable in various decision-making contexts.
Neural Networks: Deep learning, particularly neural networks, is gaining prominence in decision intelligence. Neural networks, with their ability to learn complex patterns, are applied in tasks such as image recognition, natural language processing, and other decision-centric domains.
Bayesian Networks: Bayesian Networks model probabilistic relationships among variables using a directed acyclic graph. These are valuable for representing uncertainty and causality in decision intelligence. Bayesian Networks are adept at handling situations where decisions are influenced by a combination of known and uncertain factors.
Clustering Algorithms: Clustering algorithms, such as k-means, hierarchical clustering, and DBSCAN, play a role in decision intelligence by grouping similar data points. This aids in identifying patterns and segments within the data, contributing to more informed decision-making.
Ensemble Learning: Ensemble learning methods, including bagging and boosting, combine multiple algorithms to improve overall performance. Techniques like AdaBoost and Gradient Boosting are utilized in decision intelligence to enhance the accuracy and robustness of models.
Example of AI in Decision Intelligence
Here are some examples of companies that were notable for their AI-driven decision-making applications:
Google: Google employs AI extensively in its products and services. Google’s search algorithms, YouTube recommendations and Google Maps route suggestions are examples of AI-driven decision-making applications.
Amazon: Amazon utilizes AI for various purposes, including its recommendation engine for personalized product suggestions, supply chain optimization and the automation of its warehouses using robotics and AI.
Facebook: Facebook uses AI algorithms for content recommendation, friend suggestions, and targeted advertising. The platform employs machine learning to understand user preferences and deliver personalized experiences.
Netflix: Netflix leverages AI for content recommendation. The platform analyzes user viewing habits and preferences to suggest movies and TV shows tailored to individual tastes, enhancing the overall user experience.
IBM: IBM is involved in AI-driven decision-making across industries. Watson, IBM’s AI platform, is used in healthcare for diagnosis and treatment recommendations, in finance for risk management, and in various other sectors for data analytics.
Tesla: Tesla’s Autopilot feature uses AI for decision-making in autonomous driving. The AI system processes data from sensors and cameras to make real-time decisions about vehicle control, navigation, and response to the environment.
Salesforce: Salesforce integrates AI into its customer relationship management (CRM) platform. The AI-driven features help businesses make informed decisions about sales, marketing, and customer service by analyzing customer data.
Microsoft: Microsoft incorporates AI in its products and services. Azure AI provides a range of tools and services for businesses, and Microsoft’s AI applications include chatbots, language translation, and intelligent cloud services.
ConverSight AI in Decision-Making
ConverSight AI stands as a unified decision intelligence platform featuring a state-of-the-art artificial intelligence system that is poised to revolutionize decision-making across diverse industries. Emphasizing the seamless integration of conversational capabilities and insightful data analysis. ConverSight AI introduces a sophisticated approach to comprehending and leveraging information. At its core, the system incorporates advanced Natural Language Processing (NLP), Large Language Model (LLM) and GPT algorithms, enabling it to grasp and extract meaning from human language, facilitating human-like interactions in conversations. This distinctive feature streamlines user interactions, rendering the decision-making process more intuitive and user-friendly.
Through the utilization of machine learning models, ConverSight AI delves into predictive analytics, foreseeing future outcomes by analyzing historical data and current trends. This predictive prowess empowers decision-makers to anticipate scenarios and proactively strategize for optimal outcomes. The platform signifies a noteworthy advancement in decision-making capabilities, amalgamating the potency of conversation with advanced analytics to provide users with actionable insights in real-time. This evolution marks a pivotal step in the development of intelligent, ethical and user-centric AI applications.
AI Revolutionizes Decision Intelligence
The integration of decision-making in artificial intelligence represents a transformative force across various industries. AI algorithms, ranging from sophisticated machine learning models to intuitive natural language processing systems, are playing a pivotal role in enhancing the decision-making process. These algorithms, such as decision trees, neural networks and ensemble methods, exhibit a diverse range of capabilities suited for different applications.
As AI continues to evolve, the future holds the promise of even more sophisticated decision-making capabilities. The dynamic landscape of AI-driven decision intelligence is shaping a world where human expertise is complemented and augmented by the analytical power of intelligent algorithms, leading to more informed, efficient and ethically sound decision-making processes.